More than the sum of mutations
165 new cancer genes identified with the help of machine learning
A new algorithm can predict which genes cause cancer, even if their DNA sequence is not changed. A team of researchers in Berlin combined a wide variety of data, analyzed it with “Artificial Intelligence” and identified numerous cancer genes. This opens up new perspectives for targeted cancer therapy in personalized medicine and for the development of biomarkers.
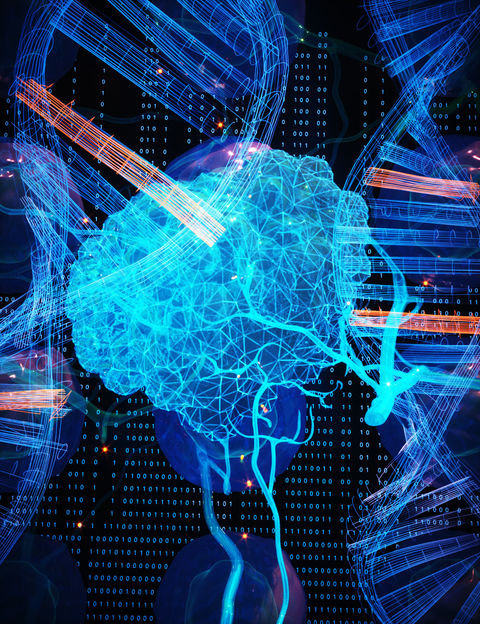
Seeing “through” the cancer with the power of data analysis – possible with the help of artificial intelligence
© MPI f. molekulare Genetik/ Ella Maru Studio
In cancer, cells get out of control. They proliferate and push their way into tissues, destroying organs and thereby impairing essential vital functions. This unrestricted growth is usually induced by an accumulation of DNA changes in cancer genes – i.e. mutations in these genes that govern the development of the cell. But some cancers have only very few mutated genes, which means that other causes lead to the disease in these cases.
A team of researchers at the Max Planck Institute for Molecular Genetics (MPIMG) in Berlin and at the Institute of Computational Biology of Helmholtz Zentrum München developed a new algorithm using machine learning technology to identify 165 previously unknown cancer genes. The sequences of these genes are not necessarily altered – apparently, already a dysregulation of these genes can lead to cancer. All of the newly identified genes interact closely with well-known cancer genes and have been shown to be essential for the survival of tumor cells in cell culture experiments.
The algorithm, dubbed “EMOGI” for Explainable Multi-Omics Graph Integration, can also explain the relationships in the cell’s machinery that make a gene a cancer gene. As the team of researchers headed by Annalisa Marsico describe in the journal Nature Machine Intelligence, the software integrates tens of thousands of data sets generated from patient samples. These contain information about DNA methylations, the activity of individual genes and the interactions of proteins within cellular pathways in addition to sequence data with mutations. In these data, a deep-learning algorithm detects the patterns and molecular principles that lead to the development of cancer.
“Ideally, we obtain a complete picture of all cancer genes at some point, which can have a different impact on cancer progression for different patients“, says Marsico, head of a research group at the MPIMG until recently and now at Helmholtz Zentrum München. „This is the foundation for personalized cancer therapy.”
Unlike with conventional cancer treatments such as chemotherapy, personalized therapy approaches tailor medication precisely to the type of tumor. “The goal is to select the best therapy for each patient – that is, the most effective treatment with the fewest side effects. Additionally, we would be able to identify cancers already at early stages, based on their molecular characteristics.”
“Only if we know the causes of the disease will we be able to counteract or correct them effectively,” the researcher says. “That's why it's so important to identify as many mechanisms as possible that can induce cancers.”
“Until now, most research has focused on pathogenic changes in the genetic sequence, i.e., in the blueprint of the cell,” says Roman Schulte-Sasse, a doctoral student on Marsico's team and first author of the publication. “At the same time, it has become apparent in recent years that epigenetic perturbations or dysregulated gene activity can lead to cancer as well.”
This is why the researchers merged sequence data that reflect faults in the blueprint with information that represents events inside the cell. Initially, the scientists confirmed that mutations, or the multiplication of segments of the genome, are indeed the main drivers of cancer. Then, in a second step, they pinpointed gene candidates that are in a less direct context to the actual cancer-driving gene.
“For instance, we found genes whose sequence is mostly unchanged in cancer, and yet are indispensable to the tumor because they regulate energy supply,” Schulte-Sasse says. These genes are out of control by other means, e.g. because of chemical changes on the DNA like methylations. These modifications leave the sequence information intact but govern a gene’s activity. “Such genes are promising drug targets, but because they operate in the background, we can only find them by using complex algorithms.”
The researcher’s new program adds a considerable number of new entries to the list of suspected cancer genes, which has grown to between 700 and 1,000 in recent years. It was only through a combination of bioinformatics analysis and the newest Artificial Intelligence (AI) methods that the researchers were able to track down the hidden genes.
“The interactions of proteins and genes can be mapped as a mathematical network, known as a graph,” Schulte-Sasse says. “You can think of it like trying to guess a railroad network; each station corresponds to a protein or gene, and each interaction among them is the train connection.”
With the help of deep learning – the very algorithms that have helped artificial intelligence make a breakthrough in recent years – the researchers were able to discover even those train connections that had previously gone unnoticed. Schulte-Sasse had the computer analyze tens of thousands of different network maps from 16 different cancer types, each containing between 12,000 and 19,000 data points.
Hidden in the data are many more interesting details. “We see patterns that are dependent on the particular cancer and tissue” Marsico says. “We see this as evidence that tumors are triggered by different molecular mechanisms in different organs.”
The EMOGI program is not limited to cancer, the researchers emphasize. In theory, it can be used to integrate diverse sets of biological data and find patterns there, explains Marsico. “It could be useful to apply our algorithm for similarly complex diseases for which multifaceted data are collected and where genes play an important role. An example might be complex metabolic diseases such as diabetes.”
Original publication
Roman Schulte-Sasse, Stefan Budach, Denes Hnisz, and Annalisa Marsico; "Integration of Multi-Omics Data with Graph Convolutional Networks to Identify New Cancer Genes and their Associated Molecular Mechanisms"; Nature Machine Intelligence